はじめに
np.polyfit, scipy.optimizeのcurve_fit, lmfitによる線形近似の実行速度の比較をmatplotlibのエラーバー付き棒グラフで表示する。
コード
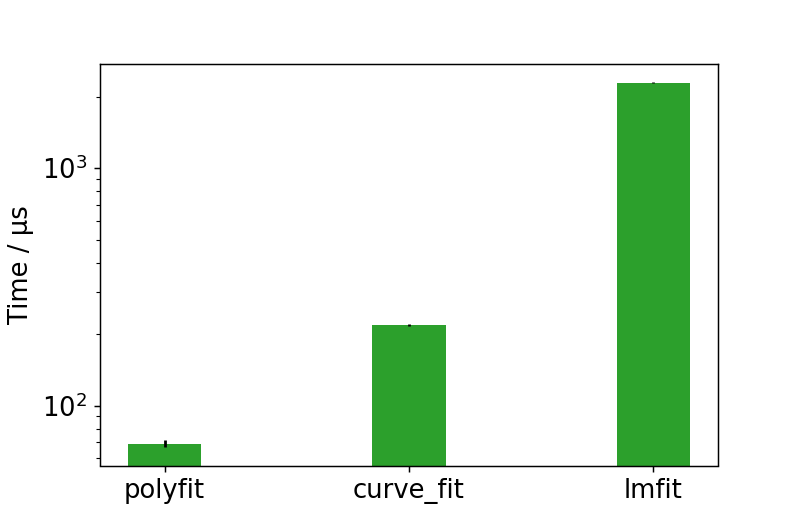
解説
モジュールのインポート
バージョン
データの作成
np.arange(0,10,1)で0から9の1刻みの配列を作成して、yのdataにnp.random.rand(10)でばらつきを付与する。
numpyのpolyfitの場合
np.polyfit(x,y,1)で1次の式でのフィッティングの係数を求める。具体的にはy=ax+bのaとbがこれでもとまる。
np.polyval(pf,x)で係数pfを使った1次の式のyのdataを生成する。
データと近似式を表示すると下図のようになる。
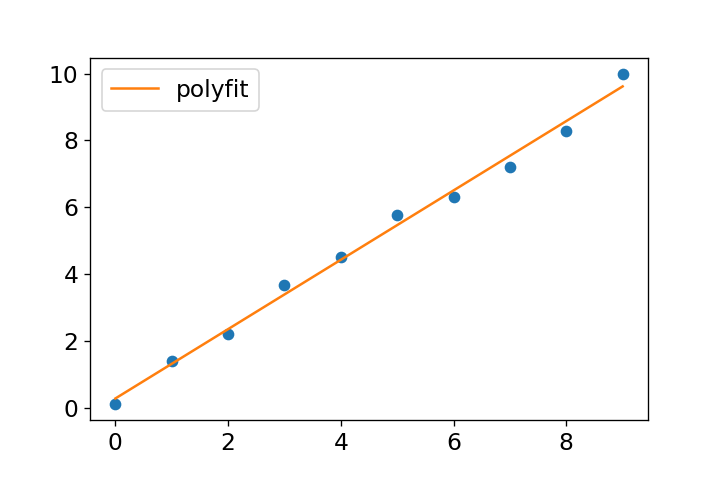
numpy polyfitの実行時間の計測
%timeitで実行時間を計測する。
scipyのcurve_fitの場合
関数を定義して、curve_fitに関数とデータと初期値を渡すことで、係数が求まる。np.polyval(popt,x)で係数poptを使った1次の式のyのdataを生成する。
データと近似式を表示すると下図のようになる。
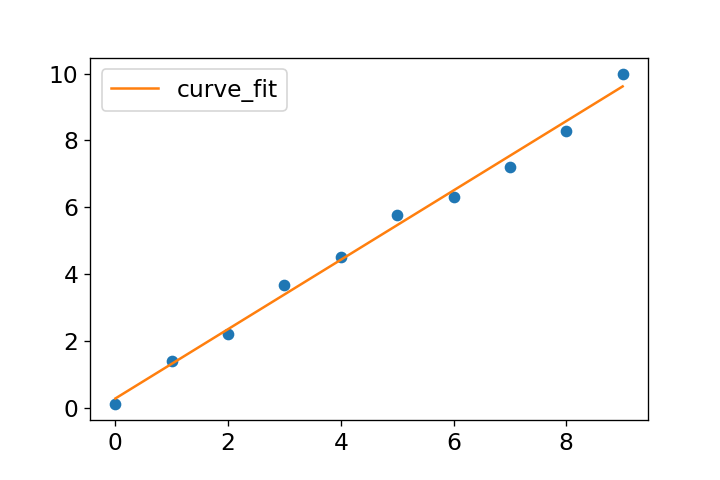
なお、決定係数の求め方については下記記事で解説した。
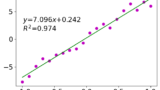
[SciPy] 1. Scipyのcurve_fitで最小2乗法近似、決定係数R2も求める
Scipyのcurve_fitの使い方と決定係数R2の求め方について説明する。
scipy curve_fitの実行時間の計測
%timeitで実行時間を計測する。
lmfitの場合
lmfitは非線形近似の高レベルなインターフェイスであり、詳細な情報を得ることができる。condaでインストールする場合は、下記のようになる。
conda install -c conda-forge lmfit
基本的な使い方はcurve_fitと同じであり、modelを定義して、初期値とデータを渡して近似を行う。近似データはresult.best_fitで得ることができる。
データと近似式を表示すると下図のようになる。
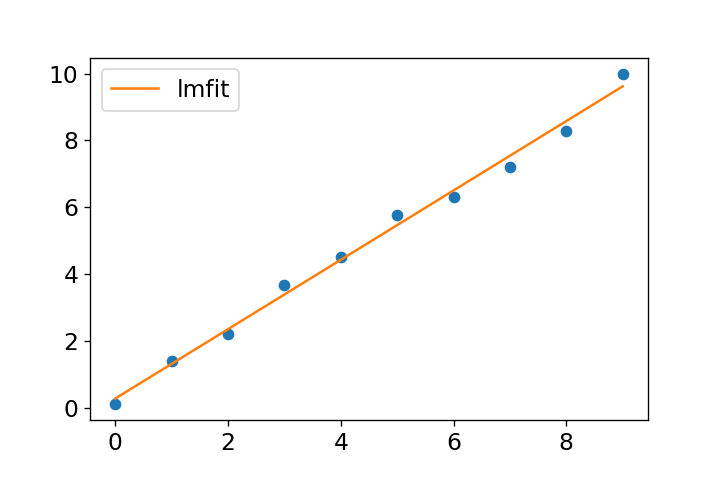
lmfitの実行時間の計測
%timeitで実行時間を計測する。
実行時間の比較
polyfitが最速で、curve_fit, lmfitの順で実行時間が長い。
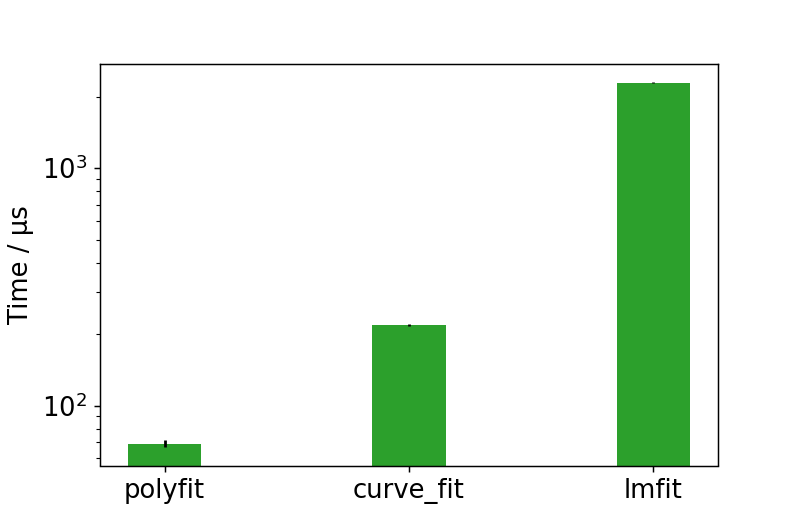
参考
numpy.polyfit — NumPy v2.2 Manual
numpy.polyval — NumPy v2.2 Manual
curve_fit — SciPy v1.14.1 Manual
Non-Linear Least-Squares Minimization and Curve-Fitting for Python — Non-Linear Least-Squares Minimization and Curve-Fitting for Python
コメント