はじめに
matplotlibのstyleの’fast’を使うことで大量データのプロットを高速化できる。ここでは、その高速化がどの程度であるかを検討する。
コード
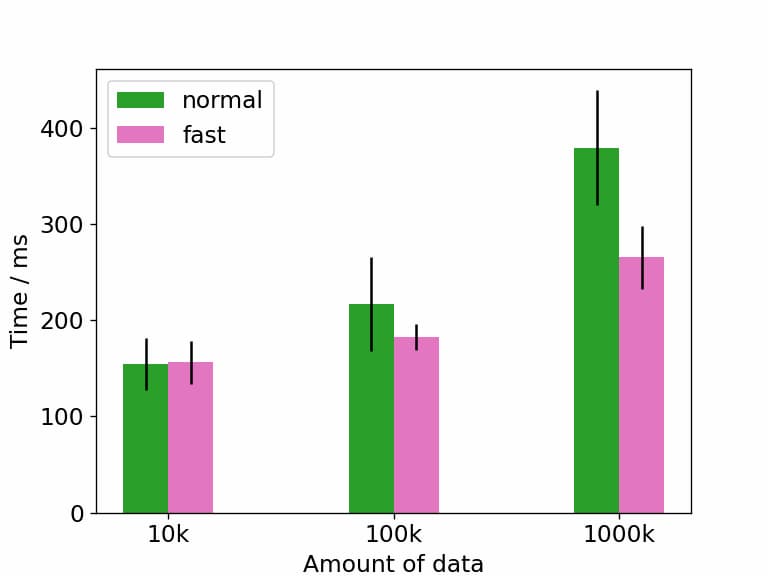
解説
モジュールのインポートなど
%matplotlibでjupyter lab, notebook内ではなく、別ウインドウで表示する。
Qt5Aggのbackendで表示する。
バージョン
データの生成
10000, 100000, 1000000 個のランダムなデータをそれぞれ作成する。
defaultスタイルでの実行時間
%%timeitでセル内のコードを複数回実行し、所要時間を計測する。
fastスタイルでの実行時間計測
fastスタイルの適用
mplstyle.use(‘fast’)でfastスタイルが適用される。
実行時間の計測
%%timeitでさきほどと同様に実行時間を計測する。
実行時間計測結果の比較
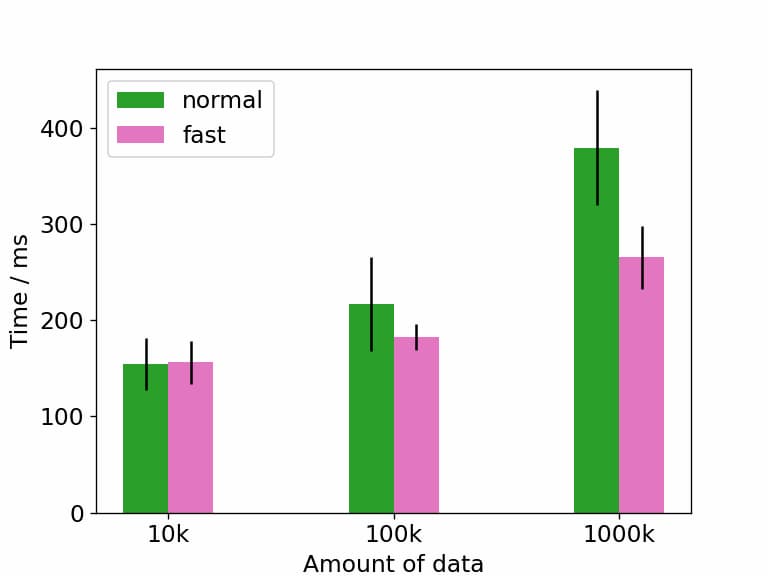
上図からfastにした際の効果は、データ数が多いほど高まることがわかる。
コードをダウンロード(.pyファイル) コードをダウンロード(.ipynbファイル)参考
https://matplotlib.org/tutorials/introductory/usage.html?highlight=fast#using-the-fast-style
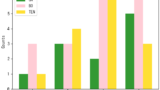
[matplotlib] 27. 横並び棒グラフ
matplotlibで横並び棒グラフを表示する方法
コメント