はじめに
ランダムな位置に出現する絵文字が拡大し消えていくアニメーションをmatplotlib, FuncAnimationで表示する。
下記記事の雨のアニメーションを参考にして作成した。
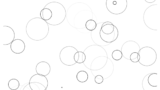
コード
解説
モジュールのインポートなど
バージョン
データの作成
numpyの構造化配列で作成する。pandasのDataFrameのような感じで配列の要素を変更することができる。
np.random.uniform(10, 20, n_drops)で10〜20の値を持つ要素数n_dropsの配列を作成する。
np.random.rand(n_drops)*20では0〜20の要素をもつ要素数n_dropsの配列を作成する。
初期データで図を表示
図中への絵文字の表示は下記記事と同様にannotateで行う。
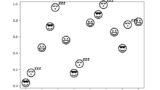
絵文字は文字列扱いなのでfontsizeでサイズを変更する。
図示すると以下のようになる。
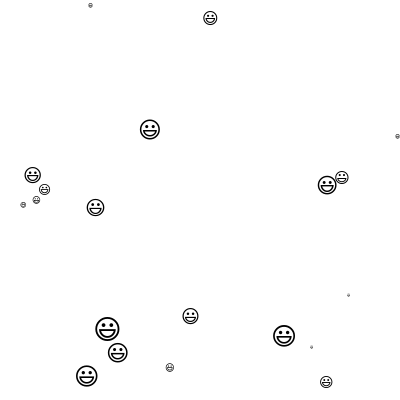
アニメーション関数の設定
current_index = frame_number % n_dropsでリセットするインデックスを決めておく。
emoji_drops[‘alpha’] -= 1.0/len(emoji_drops)でalpha値を下げていき、np.clip(emoji_drops[‘alpha’], 0, 1)で0を下回ったときに0とし、1を上回ったときに1とする。
emoji_drops[‘size’] += emoji_drops[‘growth’]でsizeにgrowthを加えていくことでsizeを大きくしていく。
78-81行ではcurrent_indexのデータをリセットしている。
ax.cla()で表示されている図をけして、すべてのデータを再びannotateで表示することでアニメーションとする。
アニメーションの表示
FuncAnimationでアニメーションを表示する。frame数はデフォルトで100なのでintervalが50msの場合、5秒のアニメーションとなる。 HTML(ani.to_html5_video())
により、jupyter notebook またはjupyter lab上にアニメーションを表示できる。
ani.save(‘ファイル名’, writer=”ffmpeg”,dpi=100)でアニメーションをMP4形式で保存することができる。
マーカーを😎とした場合
マーカーを😴とした場合
コメント